Co-authored by Premkumar Balasubramanian, Senior Vice President, Tech. & Solutions Office, Digital Solutions, Hitachi Vantara
In traditional data warehouses, specific types of data are stored using a predefined database structure. Due to this “schema on write” approach, prior to all data sources being consolidated into one warehouse, there needs to be a significant transformation effort. From there, data lakes emerge! With this transformation came the “schema on read” approach with a promise to offer flexibility in storing many data types in their native format and making them available for reporting and analytics purposes.
However, early data lakes have continued to be plagued with the problems of high complexity due to multiple systems or copies, slow performance and lack of governance (no audit trail, many formats and relatively poor access control). Business users continue to look for scalable semantic layers, analytical work benches and high-performance dashboarding capabilities.
This begs the question: Does your business ecosystem consist of disparate data environments, including data lakes and data warehouses? Do you struggle to keep data consistency across data lakes and warehouses, preventing your analysts from viewing fresh data?
Businesses today tend to struggle while maintaining legacy data warehouses and data lakes across on-premises, cloud and hybrid environments. Not only is this approach expensive, but it is also time consuming. Let us explore just how your business can benefit from building a data lakehouse.
How Can You Benefit From Building a Data Lakehouse?
What is your current data architecture?
Initially built on map-reduce, Apache Hadoop open-source data analytics platforms and other distributions, data lakes have evolved over the past decade to include object stores and run on public, private, hybrid and other cloud architectures. The data lake as a data structure is intended to bring together enterprise data and provide organizations the capability to analyze vast swathes of data. This is done through artificial intelligence (AI), machine learning (ML) and other advanced analytics that may require a wider range of unstructured and semi-structured data types. These data types may scale to much larger volumes of stored data, and often handle more complex and dynamic analytics workloads than the traditional data warehouses (see the TDWI report).
Enter the data lakehouse. This new open data management architecture combines properties of a scalable data lake with the capabilities of data warehouse, enabling reporting, analytics and intelligence across all data sources.
Cloud or On-Premises? Which Should You Choose?
The data lakehouse as an architectural construct is an ongoing reality today, especially in the cloud-native data management and analytics world. There is a clear need to support both traditional analytics as well as AI and ML workloads from the same single version of truth.
There is widespread adoption of storage and compute separation, especially in the cloud-native world and mainly from a cost-optimization perspective; those who are migrating from on-premises to cloud with re-architecture in mind are looking at this keenly. Data governance along with enabling toolsets like data catalogs, global metadata, master data management or data quality (MDM/DQ, with emphasis on Big Data) are being reconsidered anew. All of this has led to the emergence of data lakes that store any and all data in one place, allowing both reporting and analytics capabilities, while providing the governance required to manage the data.
The Data Lakehouse: Key Advantages
Improve Efficiency and Cost
The advantages of building a data lakehouse include: lower cloud costs, since you are eliminating costly data warehouses; faster ad-hoc queries, reporting and dashboards; and the simplicity of use. Cloud data lakes provide data analysts and business analysts with simple and easy access to datasets directly from the cloud. Regulated industries are gradually adopting cloud without compromising on the data privacy regulations through hybrid environments.
Add Scale, Speed and Availability to Your Business Data
A large library of integrations, transformations and blueprints allows businesses to provide elasticity, scalability and flexibility in data lake deployments. The composable data lake allows the ease of adding or removing services from public cloud vendors and third-party software packages.
Businesses can also benefit from reduced data movement and redundancy while spending less time and effort administrating the datasets involved. Therefore, a data lakehouse makes all data available to the entire organization, and specifically to the analysts who use it.
How Hitachi Can Help
There is a considerable proportion of large on-premises enterprise data warehouse (EDW) investments still in existence. However, their migration to, re-architecture and coexistence with the modern data lake house type of architecture (especially cloud-based) are accelerating rapidly. Combining with a data fabric as a unified data mesh for enterprise data integration addresses need for real-time data ingestion coexisting with other batch or microbatch workloads, resulting in Lambda architectures. This is more or less a settled approach in cloud-native data ingestion scenarios today.
Hitachi Vantara’s solution for cloud data lake modernization delivers data and analytical model management, governance and self-service capabilities. Our secure, production-ready data platform can be quickly and efficiently deployed in hybrid, cloud or multicloud environments. Combining our experience with industry blueprints and automation-based accelerators, we have completed hundreds of cloud transformation programs, helping enterprises to increase business agility and improve the resilience of their IT landscapes.
As enterprises turn to data and analytics to power their business models, the need for creating digital organizations is skyrocketing. Hitachi Vantara helps build, secure and manage your data lake; we clean and deduplicate data using machine learning to improve data consistency and quality.
We provide the resources and tools that allow you to manage and modify your data lake as your requirements grow. The large selection of modular open-source and Hitachi software as well as cloud service provider-aided capabilities, allow organizations to create specific pipelines to solve individual use cases. Then our integrated solution enables effective management and security of the datasets, while allowing various forms of published data to assist different business requirements.
George Philip is Director of Consulting Services at Hitachi Vantara.
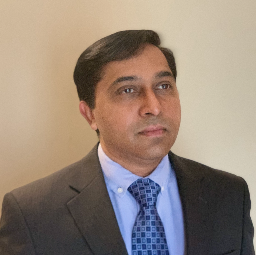
George Philip
George is responsible for developing and implementing solutions spanning modern cloud-based big data lakes and lake houses, AI/ML, IoT, data engineering and visualization for clients across industries including financial services, manufacturing, retail, CPG and government.