Modern Data Foundation for AI-Driven Results
The following is Part I of a three-part series.
In our modern digital society, data is abundant, and storage is affordable. Businesses, governments and even individuals can (and do) collect every transaction, click, swipe, location, message and attribute in their datasets. With just a few clicks on my smart device, I can review data on every place I’ve been, how much I spent, every step I took, what the weather was like and who I was with. Businesses collect the same abundance of data. However, are we getting the benefit and insights from what’s collected? Not really. The apps on my phone allow me to see the data in a somewhat structured format, but I’m still not always sure what to make of it. Years ago, Professor and author Thomas H. Davenport and team were telling us in the Harvard Business Review that less than 1% of unstructured data gets used by organizations.[1] Still today studies have found less than two-thirds of all data available to enterprises gets leveraged at all, much less leads to insights[2].
We know data has a profound ability to improve quality, save money and resources, and change the world. A few, triumphant big tech companies have harnessed the power of data, but why is it so difficult for the rest of us to gain beneficial insights from the data being collected?
- There is no lack of smart ideas: When I’m brainstorming with clients, keen hypotheses and big business opportunities fill the room. Managers, executives and technicians can and do identify endless variables and analyses with the potential to lead to game-changing insights.
- There is no lack of data: I rarely hear “I wish we had xyz data” when I’m meeting with clients. They know the data exists and they often already have the data in house.
So where is the disconnect? How can organizations bridge the gap? Here’s part one of three areas I will discuss as part of a series to help you drive with analytics.
Prioritize Data Accessibility
The right data needs to be available to the right people, at the right time. While this may seem obvious, it’s a struggle for many organizations to implement, for multiple reasons.
- Every department is driven by its own goals for the data, driving different cloud choices, and becoming very siloed. Each department uses different governance, storing and tagging of data, which makes it harder for analysts and scientists to get a holistic view, much less a normalized one.
- Data architectures are simply getting very complex: Each component of today’s data pipelines takes a good deal of configuration, driving a need for specialized tuning and skills owned by siloed departments. Ultimately, it becomes difficult to figure out what the data was even for in the end. A considerable number of today’s enterprise data architectures are duct-taped together: traditional with modern data solutions. They are not designed to work together across the edge-to-core multicloud pipelines.
- Siloed, nonsynergistic, architectures lead to encrypted data within each business unit. Decryption and access across silos becomes time consuming, complicated and code heavy.
To address these challenges, here are actions to consider:
- Spend time creating a streamlined, end-to-end data architecture — one that is supported by intelligent tools and accommodates needs of your stakeholders.
- Throughout the data life cycle (discovery, ingestion, cataloging, tagging, storage, security, governance and access), ask the question: “Will this make the data easily available for analytics?” It may be tempting to build the data architecture with seemingly cheap, disparate open-source tools. However, the savings will be negligible in the resource costs needed to maintain and decrypt the nonsynergistic systems. Start with the goal to get the correct data to the analyst — quickly, efficiently and securely — not to build the most inexpensive architecture.
- Rather than having any given component be evaluated from an individual business unit’s needs, consider asking: “What problems is this data solving, and what problems can it potentially solve for the organization?” Identify interdependencies for the data to be accessed then resolve and tag them appropriately, removing coding and access constraints where possible. This will allow for your data analysts to have easier access to the data as and when they need it, with fewer decryption barriers.
Even mature companies with powerful architectures that can ingest a lot of data, fast, with strong governance and data management, can have trouble getting data into the analysts’ hands quickly and easily. We at Hitachi recently worked with a financial services client to see what was slowing down their time to insights. This client had carefully designed governance and data management processes and was successfully landing millions of highly secured files of data a day. However, the processes were cumbersome, delaying the data availability, and the data was abstracted from end users, making it hard to navigate.
To create the solution, we started by asking the how the firm intended to use the data to drive the business. The result was an API-driven, automated cataloging process, to reduce the time to data availability, and a user-friendly, self-service “marketplace” data-catalog user interface (UI), to reduce complexity in navigating the data.
It’s also typical to see that clients have siloed and redundant data, which makes it hard for the analysts to find trustworthy, valuable data. A global organization had a costly and inefficient data architecture that made it unwieldy for the analytics teams to do their work. This Hitachi client had 25 relational data-warehouse siloes, all being managed separately, to meet the needs of 25 different business units. Hitachi helped them complete a data transformation project to consolidate their data into one cataloged data lake and removed data redundancies. Now, analytics projects move faster because there’s no need to forage for data. It’s all in one place. Costs were also reduced, as the company’s data footprint was consolidated down from 4PBs to hundreds of terabytes.
Again, always ask: “How do you intend to use the data, who will need it throughout the process, and can they get to it in timely fashion and in a secure way.”
In part two, I will discuss tips on how to leverage automation for governance. Until then, be safe, be healthy, be data driven!
Read Part Two: Leveraging Automation Technologies for Data Governance
[1] Harvard Business Review, “What’s Your Data Strategy?” featuring Tom Davenport, April 18, 2017.
[2] Frontier Enterprise, “Two-Thirds of Data Available to Firms Goes Unused,” July 2020.
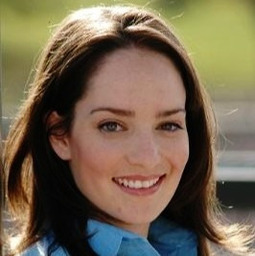
Miranda LeQuire
Miranda has +15 years' experience driving and realizing strategic goals via data and insights for a range of companies and business units, from start-ups to global F500 to technology consulting. As well as in marketing, sales, HR, strategy, finance and IT.