In financial services, data has always been viewed as a strategic asset. To manage this data, organizations have invested heavily over several years and across a number of technology generations in the underlying data infrastructure. This approach has left a large data technology legacy along with silos of data linked to specific infrastructure and applications.
Data-Led Strategy
As financial services organizations look to embrace much broader data-led strategies, they are combining both transactional and nontransactional data with advanced machine learning (ML) algorithms, to drive intelligent capabilities across all products and services.
The key challenge facing organizations that are developing these advanced products and services is to evolve their data infrastructure. They need to enable larger computational and data resources, which are required to run algorithms that underpin analytical methods. These methods are foundational to future of digital financial services.
Evolution of Data Architecture
This new generation of digitized products is driving adoption of the high-performance data infrastructure that is needed to enable new capabilities. This trend is amplified with the wide-scale adoption of cloud technologies, which drive an evolution from specialized data infrastructure to a cloud-native architecture. This architecture can support advanced analytics, ML and deep learning within a single environment.
This new course in data architecture will provide a number of key foundational capabilities that enable a converged approach, which is based on:
- High-performance data architecture. This reduces the need for complex data transformations and pipelines, which allows for data analytics to be performed directly on in-place data. Leveraging highly parallelized compute and storage enables data to move seamlessly across the environment using high-speed in-memory interconnects.
- Separation of compute from data. This provides the ability to reduce data movement and duplication by substituting real-time computation and high-performance data infrastructure. It reduces complexity of data pipelines and increases data agility.
- Shared data platform. This supports analytics, ML and deep-learning analytical activities on a single open data architecture. Different methods can be employed across the platform, such as advanced data analytics, data science tooling, modern data pipelines and data management frameworks — all within a single shared data environment.
Historically, these types of analytical and ML activities have been viewed as separate technology stacks. This has created complexity and has had a significant impact on an organization’s ability to be agile and innovate.
As demand for these new capabilities increases, we expect to see converged analytics architecture becoming the default approach for large financial services organizations as there are significant benefits in adopting a common data platform:
- Hybrid or public multicloud deploy-ability allows support for both static and dynamic deployment models, enabling a variable versus fixed cost of operations.
- Open standards and open-source technologies are used extensively, reducing lock-in and cloud-provider dependencies.
- Simplification of data architecture provides a common platform for all classes of data, creating the opportunity to reduce costs through system rationalization and operational costs efficiencies.
Converged Analytics
Beyond operational, technology and cost benefits, it’s clear that financial services organizations choosing to adopt a converged analytics strategy will be in a position to realize significant competitive advantage: The platform will provide a foundation for creating highly differentiated data-driven capabilities:
- Converged analytics provide a comprehensive set of integrated analytical, ML and deep learning frameworks, which can be consumed on an on-demand basis. This approach allows for analysts, data scientists and data developers to use shared data with different analytical and ML models.
- Fast data enables high-speed, lake-house-type data repositories that provide standard business intelligence access mechanisms, as well as high-performance in-memory access for more computation-intensive data applications.
- Agility allows fast, agile data development cycles with fully integrated development and production data pipelines that use modern DataOps approaches to drive continuous iteration of digital products and services.
Financial services organizations are competing to create the next-generation data-driven digital products. To be successful in this changing competitive landscape, organizations that want to lead will need to reformulate their data architectures and look to establish a converged analytics strategy.
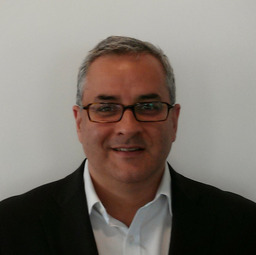
Thomas De Souza
Thomas is a multidisciplinary technologist with an eye for business model innovation and leading-edge data technologies. He has +20 years' experience in financial services with Booz & Co, PwC, a VC-backed FinTech, and global banks including JP Morgan, Deutsche Bank and Citi.