This is the third story in the “Our GenAI Journey” series.
One of the unspoken truths about generative AI (GenAI) is that everyone is learning as they go. Perhaps that’s because the pace of innovation is so quick that the learning curve continues to soar upward.
Consider even the most recent advances, including Microsoft’s plans to integrate Chat GTP-4 Turbo into Copilots to handle “complex and longer tasks”; Google’s rollout of the Gemini suite of models Ultra, Pro and Nano that support everything from high-end hardware to mobile phones; and even Apple’s release on GitHub of its ML-Explore (MLX) array framework for machine learning designed for Apple silicon.
The pace can seem dizzying at times. We at Hitachi Digital Services along with our parent company, Hitachi Ltd., have been working in AI for decades and even we are learning new things about the capabilities, opportunities, and potential risks of GenAI every day.
In fact, if someone from a cloud provider, systems manufacturer, software developer, etc., tells you they have it all figured out, be cautious. The technology is advancing so quickly that very few have all the answers on any given day. On the other hand, if they listen and commit to working with you, partnering with you, to solve your problems and achieve your goals with the help of GenAI, they’re worth considering.
I’m intimately familiar with this space and with GenAI and AI development. In my role as the Chief Technology Officer of Hitachi Digital Services, I work directly with large global customers to help them overcome challenges and achieve business goals through digital solutions. Increasingly, and not surprisingly – as my colleagues have stated in the previous two stories – the advent of GenAI and AI pose some of the most dramatic opportunities for companies, as well as some significant risks. It’s critical to engage with the technology, but in the most thoughtful manner possible.
In my line of work, we like to architect solutions and, wherever possible, begin to template certain aspects of them for rapid reuse in other, comparable, customer challenges. But GenAI has changed this philosophy in a big way, mainly because these kinds of solutions are geared for specific workloads, for specific customers in specific industries with ultra-specific parameters. In other words, very little of these ‘snowflake’ type solutions can be packaged up and reused.
One pattern, however, that has emerged with the rapid adoption of GenAI is in the levels of customer understanding. I’ve recognized the savvy, the less savvy, and the unsavvy but eager organization.
Interestingly, often what separates these three categories is not the challenge or choice, but the severity or scale.
Making the Critical Decisions
For example, every organization must understand early on the basic choices that await them with GenAI.
What engine should I use? Is on-premises a better option for me than doing this work in the cloud? (And, if I go to the cloud, do I risk becoming a hostage to the cloud provider?)
Then the discussion turns to the brass tacks of AI and which type of large language model (LLM) should be employed. Smaller models like LLaMA 2 models are trained on 7 billion to 70 billion parameters and are well suited to run on-premises or in the cloud, while OpenAI’s GPT-3 and GPT-4 are trained on between 175 billion and 1 trillion parameters, and typically are better used with the compute power afforded by the cloud.
As I stated in a recent story, some of the things to consider when determining whether a local LLM and an on-premises footprint may be more beneficial than leveraging public cloud include, but are not limited to, the training frequency and training data.
For example, as my colleague at Hitachi Vantara, Bharti Patel, recently wrote, her GenAI work at the company led them to build their own system on-premises to support their work with LLaMA 2. Among the reasons for the move, she said, was for greater control of the data and management of the LLM.
The Customer Angle
These are only some of the varied issues and decisions I see customers wrestling with every day. For example, as I alluded above, increasingly security, data privacy, and issues surrounding bias in models are of critical concern. One global bank we worked with was intent on eliminating the risk of its GenAI bots from producing responses/results or responding to queries with unparliamentary, or offensive language.
We went to work and applied several leading technologies directly from prompt engineering, which is the process of writing natural language text instructions for GenAI models, to responsible AI (i.e. toxicity analysis, hallucination controls, etc.). In particular, we applied retrieval augmented generation (RAG), an AI technique that combines information from external datasets allowing AI models to retrieve relevant information from a knowledge source and incorporate it along with the generated text.
Working with these technologies and tools, we built a program, called AI Compass, that measures an AI model across different parameters such as sentiment, toxicity, potential jailbreaks, refusals, etc. This is extremely critical as companies move from proof-of-concepts to production GenAI use cases.
And it was more than appropriate for the bank, because it needed more than just toxicity analysis – it needed to ensure all locations were compliant and consistent in their responses across the multiple dimensions mentioned above.
Manufacturing Opportunities
Sometimes, customers believe they need a tool or technology, when after assessment, another approach is far more effective. In another GenAI instance, a leading manufacturer of residential home products wanted to leverage the tech to help the company better automate its extremely complicated pricing system. To the uninitiated, a lot of remodeling products have dependencies when it comes to pricing. From custom sizing to the myriad types of materials available, from regionality to seasonality, every part proposed comes with a number of challenges to overcome before recommending a fair price. What the company craved was a way to automate this process. And the truth was, they had a lot of data to leverage, a lot of historical data, but needed a way to generate answers quickly to close more sales.
After going over the challenge with the company we all realized that this was not merely a Gen AI use-case. Rather, it was a great opportunity for more traditional machine learning and prescriptive automation that could then be wrapped with GenAI for easier consumption by customers. So, we have embarked on a journey with the company, with my group at Hitachi Digital Services working alongside data scientists and engineers from Hitachi America Limited to scope and execute.
If our work in AI and GenAI has taught us anything, it’s that we’re all in this together, with our customers and partners, and within our own groups. We’re all learning fast together and moving forward. Our Executive Chairman, Gajen Kandiah, said it best in a post not long ago when he encouraged readers not to delay in engaging with GenAI. Begin projects, experiment, but do so with guardrails, thoughtfully.
Related
· Tracing Our First GenAI Steps
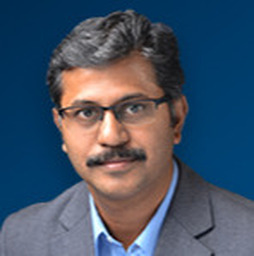
Premkumar Balasubramanian
Prem leads innovation for Hitachi Digital Services, with responsibility for strategy and support of GTM pursuits. Including repeatable customer solutions and thought leadership focused on cloud, data, IoT and GenAI..He previously held a similar position at Hitachi Vantara.